
GCPEx: Measuring Frozen Precipitation from Space
Frozen precipitation is particularly difficult to measure from space due to the wide variability in snowflake shapes and behavior. Snowflakes can have different impacts on the active and passive instruments signals compared to liquid precipitation, which is further complicated by a weak signal to noise ratio resulting from different scattering properties of liquid verses frozen precipitation.
In recent years, the capability to quantify liquid precipitation from space has been greatly enhanced with the addition of several measurement capabilities from low-Earth orbit, most notably from passive microwave (PMW) sensors such as the Tropical Rainfall Measuring Mission (TRMM) Microwave Imager (TMI), Earth Observing System (EOS) Advanced Microwave Scanning Radiometer (AMSR-E) onboard Aqua, the Defense Meteorological Satellite Program (DMSP) Special Sensor Microwave/Imager (SSM/I), Windsat onboard the Coriolis satellite, and the SSM/I-Sounder (SSMI/S) onboard DMSP F-16. These sensors commonly referred to as imagers, estimate the vertical hydrometeor profile using top-of- atmosphere (TOA) based on observed upwelling brightness temperatures (TB) at 6, 10, 19, 37, and 85 GHz.
PMW sounders, such as the National Oceanic and Atmospheric Administration (NOAA) Advanced Microwave Sounding Unit (AMSU-B) and AMSU-B’s next- generation sensor the Microwave Humidity Sounder (MHS), offer an alternative for detecting and estimating falling snow based on higher frequency measurements ranging from 150 to 183 GHz. Sounders have the advantage of being sensitive to the ice water path (IWP), (but not necessarily the surface snow) in the cloud and less sensitive to uncertainties in prescribed surface emissivity.
Each of the state-of-the art precipitation sensors has characteristic strengths and weaknesses. This suggests that, despite recent advances in sensor technology, there remain several key areas of space-based precipitation retrieval that are not well understood and their impacts are especially acute at higher latitudes. A large portion of the global population resides at latitudes where a significant fraction of the fresh water originates from falling snow or snowpack melt. In fact, falling snow contributes up to 80% of the precipitation occurrence in Polar Regions. Therefore, there is a great motivation for improving snow precipitation retrieval algorithms and resolving discrepancies between products from different sensors.
Unfortunately, there is little or incomplete validation data for frozen precipitation. Since the majority of precipitation events at high latitudes are associated with spatially incoherent, shallow cloud layers which produce light but persistent snow (and a significant accumulation), detection limitations of current sensors may lead to a substantial underestimate of accumulated snow and its contribution to the associated water cycle. It is, therefore, important to both characterize the abilities of current satellite precipitation sensors to detect and estimate the intensity of frozen precipitation events and an accurate characterization of the microphysical properties of such events upon which to base development of new algorithms for future sensors such as the DPR and GMI that will fly on the GPM Core Observatory.
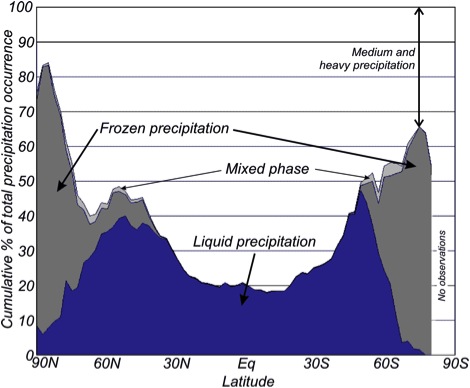
The GPM Core Observatory, which is scheduled to launch in February 2014, will carry the first space-borne Ku/Ka-band Dual-frequency Precipitation Radar (DPR) and a multi-spectral (10 to 183 GHz) GPM Microwave Imager (GMI). The GMI is designed with special attention to instrument accuracy and stability to serve as a reference for developing a transfer standard to unify radiometric measurements by a constellation of microwave sensors. The increased sensitivity of the DPR relative to the TRMM radar and the high-frequency channels on the GMI will give GPM new capabilities relative to TRMM to take on the challenge of measuring light rain and falling snow, which account for large fractions of precipitation occurrences outside the Tropics, especially in winter seasons over land.
Next Topic: Cloud Resolving Model (CRM) Simulations